Stellar Performances: How CrowdStrike Machine Learning Handles the SUNSPOT Malware
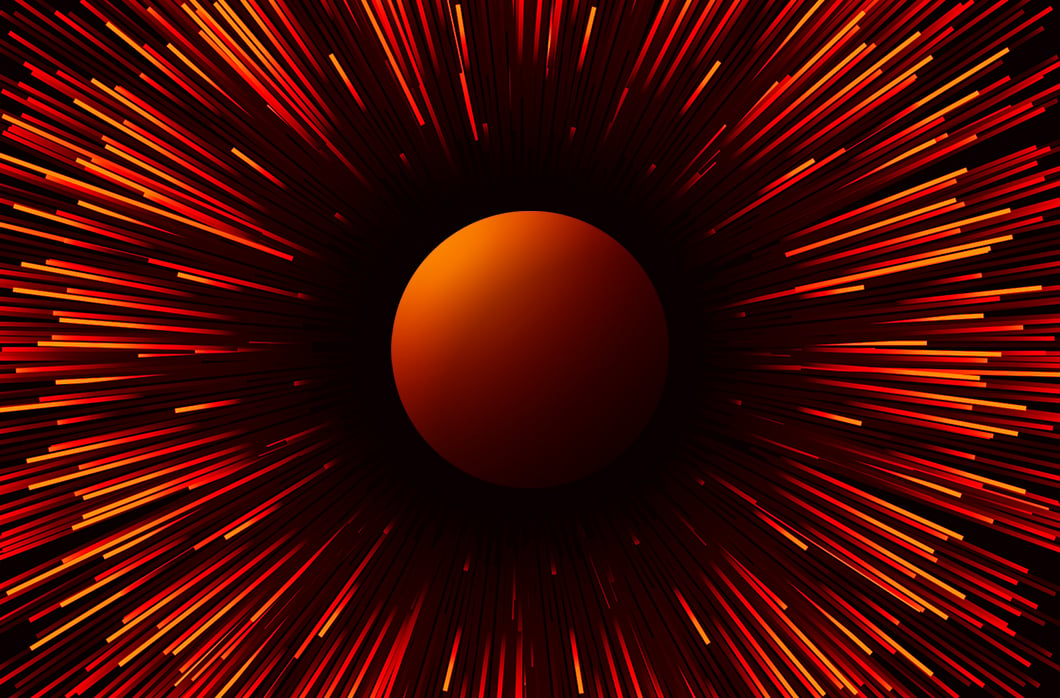
The CrowdStrike® Intelligence team recently published its findings on a sophisticated supply chain attack. In a nutshell, the adversary planted a malicious file, dubbed SUNSPOT, on the victim’s build system. SUNSPOT then monitors when new software is compiled and inserts a malicious payload clandestinely during the build process.
Such targeted attacks are normally the domain of indicators of attack (IOAs), which detect illicit behavior by observing the actions and the intent of processes on endpoints. But besides IOAs, CrowdStrike Falcon® PreventTM leverages other techniques for threat detection, including file-based machine learning (ML).
The main component of SUNSPOT is a file taskhostsvc.exe
with SHA256 hash
c45c9bda8db1d470f1fd0dcc346dc449839eb5ce9a948c70369230af0b3ef168
. The file’s compile timestamp indicates that the file was compiled on February 20, 2020. While this data field can be easily manipulated, we speculate that the adversary did not go through this effort as it aligns with the timeline for the rest of the attack.
To check how well our file-based models pick up on this thread, we ran the file against the on-sensor ML model that we shipped in September 2019, about five months before the file was presumably created. It was detected at high confidence.
While one should not rely solely on static analysis-based techniques, especially for sophisticated attacks such as this one, it validates the power of signature-less ML models that can detect threats based on generic properties as opposed to the reliance of a human analyst creating a suitable signature.
Additional Resources
- Read the blog “SUNSPOT: An Implant in the Build Process.”
- Read a blog on the SUNBURST attack from SolarWinds.
- Download the CrowdStrike 2020 Global Threat Report.
- To learn more about how to incorporate intelligence on threats into your security strategy, visit the CROWDSTRIKE FALCON® INTELLIGENCE™ Premium Threat Intelligence page.
- Get a full-featured free trial of CrowdStrike Falcon® Prevent™ and learn how true next-gen AV performs against today’s most sophisticated threats.