Modern cybersecurity is a game of speed. With attacks now happening in mere minutes, the agility with which security teams can detect and disrupt adversaries can make the difference between being the hunter or being hunted.
However, reclaiming a speed advantage against adversaries can be a daunting task for security teams. Today’s defenders must grapple with unfilled security roles, crippling complexity and unrelenting alert volume — all of which aggravate response times and leave teams wasting minutes on non-urgent tasks. All the while, adversaries are climbing to new heights of sophistication and stealth, increasingly propelled by AI and automated technologies.
To ensure teams can stop breaches faster and more effectively than ever before, CrowdStrike is announcing four groundbreaking AI innovations to elevate and expedite each stage of the analyst experience. These additions will enable frictionless data ingestion, reimagine alert prioritization, visualize potential attack paths and accelerate detection triage. Each innovation harnesses AI to shatter persistent barriers to effective threat response including manual data onboarding, frenetic and unprioritized alerting, overlooked blind spots and error-prone decision-making during incident triage.
Frictionless Data Ingestion with Industry-first AI-generated Parsers
One of the biggest challenges security teams face arises before they even start analyzing detections, beginning with data onboarding. Security teams are drowning in data from countless novel sources, each with its own unique log format. Developing custom parsers for these logs can be a time-consuming, resource-intensive process, especially as formats constantly evolve. Keeping up with these changes while ensuring data remains accurate and accessible is a persistent struggle, often leading to deployment delays, increased costs and overburdened teams.
CrowdStrike Falcon® Next-Gen SIEM is changing the game with AI-generated parsers, which enable SOC teams to quickly ingest and process data from any source. Instead of painstakingly writing parsers and manually updating them every time log formats change, teams can leverage the power of generative AI to build parsers instantly based on representative logs.
To generate parsers, users simply provide sample log records. From there, Falcon Next-Gen SIEM analyzes the samples with multiple large language models (LLMs) to learn their structure and log content. AI-generated parsers adhere to the CrowdStrike Parsing Standard, ensuring seamless correlation and enhanced threat detection.
By dynamically building parsers, security teams can eliminate hours of busywork and accelerate deployment of Falcon Next-Gen SIEM. Discover the latest innovations that ease data onboarding for effortless legacy SIEM replacement.
Proactive Exposure Management with AI-powered Attack Path Analysis
Another persistent issue security teams face arises in proactive exposure management workflows. Faced with thousands of vulnerabilities and limited resources, prioritization is a challenging yet crucial task for security teams. Traditional vulnerability management tools often prioritize vulnerabilities based on individual severity scores, which don’t provide sufficient context to effectively prioritize: Over 50% of all CVEs are rated as either high or critical in severity based on CVSS scores, offering limited information to work with. These scores also ignore the environmental context of the organization’s asset landscape, which informs exploitability.
Delivered through CrowdStrike Falcon® Exposure Management, CrowdStrike’s Attack Path Analysis (APA) addresses these issues with predictive AI and machine learning technology. It enhances vulnerability severity rating through ExPRT.AI, CrowdStrike’s proprietary technology trained on real-time threat intelligence and billions of detection events daily. ExPRT.AI predictively and dynamically evaluates a vulnerability’s exploitability to narrow down the percentage of true critical issues teams need to focus on. Machine learning algorithms help detect asset roles in order to inform an asset’s business criticality, whether it’s a jump host, email or web server, providing important environmental context.
Armed with this information and deep insight from the CrowdStrike Falcon® platform, APA maps out how attackers could intrude and move laterally through the customer’s environment to compromise critical systems. It distinguishes real from theoretical attack paths and identifies choke points or dead ends so teams can uplevel their defenses to proactively seal off paths.
What’s more, CrowdStrike’s APA is capable of mapping cross-domain interconnections between cloud and on-premises assets, matching the hybrid infrastructure reality most customers live in, and highlighting predictors of attack based on traditional CVEs and cloud-native misconfigurations. It also delivers prioritized, high-impact, low-effort remediation recommendations so teams can act quickly, facilitating targeted response — especially in the case of zero-days, when minutes matter. As the saying goes, attackers think in graphs, defenders think in lists. With APA, defenders can now visualize the attacker perspective in order to get ahead.
Automated Leads Powered by CrowdStrike Signal
Yet another prioritization challenge arises when security teams need to find the “needle in the haystack” — surfacing the detections that most urgently warrant analyst attention. Traditional detection systems often struggle to identify complex threats and can overwhelm analysts with numerous unprioritized alerts. These legacy approaches frequently apply generic rules and thresholds that fail to account for the unique characteristics of each environment. Without AI assistance, analysts must manually triage and piece together disparate information, leading to alert fatigue and the risk of missed threats.
CrowdStrike Signal, a new AI-powered engine for CrowdStrike Falcon® Insight XDR, addresses these challenges by generating and intelligently prioritizing automated leads. By grouping related events into actionable insights and providing a clear starting point for investigations, CrowdStrike Signal reduces noise, accelerates detection and response, and ensures security analysts of all skill levels can quickly identify and neutralize threats.
CrowdStrike Signal enhances early threat detection by analyzing a broad range of data, including subtle and early-stage indicators, allowing security teams to identify and respond to potential threats before they can cause harm. With Signal’s AI-driven approach, detection adapts to the specific characteristics of each environment. This ensures only the most relevant and critical threats are surfaced for human validation, enabling more accurate prioritization and response. By adapting to your organization and providing an at-a-glance measure of its current posture, Signal reduces the likelihood of missed threats and enhances overall security.
Accelerated Response with GenAI-led Detection Triage
A final challenge security teams face is in expediting triage and response capabilities. To further compress investigation and response times (MTTR), security teams will be able to invoke CrowdStrike® Charlotte AI™ to accelerate detection triage, expediting the most time-consuming, error-prone components of initial triage: discerning between true and false positives and reporting their assessments. This feature will enable Charlotte AI users to apply the world-class detection triage guidance of CrowdStrike experts across incoming detections with the speed, consistency and scale of AI. As with all actions enabled by Charlotte AI, users will be able to configure upfront which classes of detections this feature can be applied to and use CrowdStrike Falcon® Fusion SOAR to decide what actions can happen based on the AI’s findings.
When a new detection is issued, Charlotte AI will first analyze the detection to determine whether it is a true or false positive and provide an associated confidence level for its assessment. Charlotte AI will also recommend whether the detection should be closed or referred to a human analyst. During this triaging phase, analysts will be able to make Charlotte AI’s escalation decisions a condition in Falcon Fusion SOAR workflows to automatically notify analysts when to begin a forensic analysis or investigation. Finally, Charlotte AI will generate an explanation summarizing its findings and recommendations (see figures below).
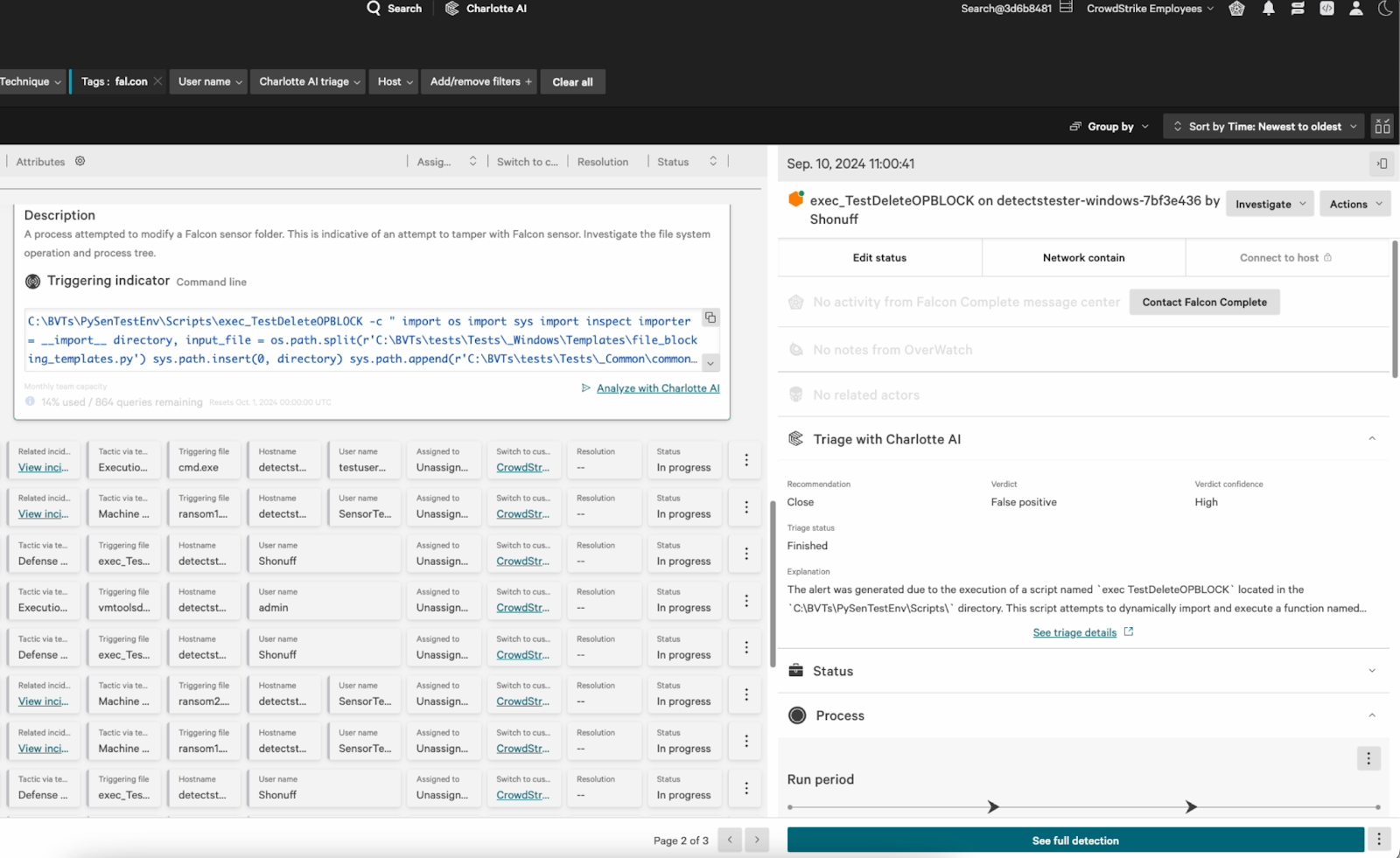
Figure 2. Charlotte AI determines that a detection is likely a false positive (non-malicious), recommending the detection be closed and explaining its recommendation. (Click to enlarge)
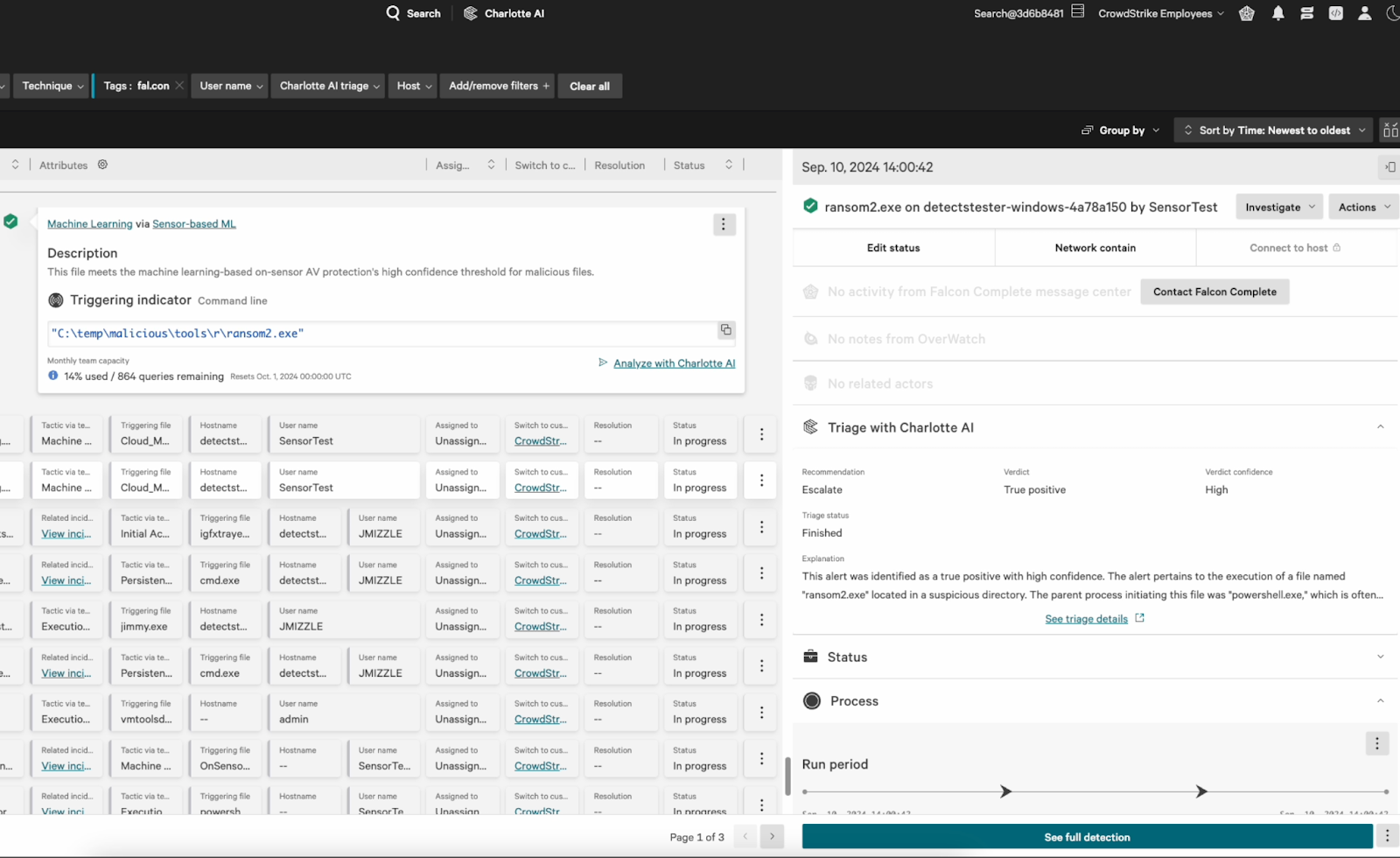
Figure 3. Charlotte AI determines that a detection is likely a true positive and recommends escalation to an analyst for further investigation. (Click to enlarge)
Next Steps
- Learn more about what’s new at Fal.Con.
- See the latest from Fal.Con, including keynotes and live-streamed sessions.
- Attending Fal.Con 2024? Read more about the AI sessions we’re most excited about.